TL;DR:
- Enterprises see big potential in both open-source and commercial LLMs for generative tasks, information extraction, text classification, Q&A/search, and personalization
- Data privacy, cost, and customization complexity are the top challenges in deploying LLMs
- Open-source LLMs are rising in popularity, enabling more privacy and customization compared to commercial offerings
- To prepare for LLMs, establish use guidelines, train employees, break down data silos, and consider leveraging an LLM platform
- LLMs will likely unbundle and augment knowledge work more than fully replace jobs in the near-term
A survey of 150 enterprise leaders provides an inside look at how organizations are adopting large language models, the opportunities and obstacles they see, and what it means for the future of work.
Will Large Language Models Really Change How Work Gets Done?
Large language models (LLMs) have taken the world by storm. Propelled by the launch of OpenAI‘s ChatGPT, a new LLM company seems to be born every day, and bigger, better open-source models are released weekly. With all these options, enterprises are scrambling to determine how to gain a competitive edge with these powerful new AI capabilities.
But are LLMs all hype, or will they really change how work gets done? Predibase surveyed 150 executives, data scientists, developers, and other leaders to find out. The results paint a picture of immense potential–but also noteworthy challenges that organizations need to navigate.
LLMs Have Remarkable Potential Across Business Functions
When it comes to LLM use cases, most minds immediately jump to chatbots and generative content creation, thanks to ChatGPT’s popularity. Indeed, the Predibase survey found generative AI is the top use case, with 35% pointing to applications like summarizing content, writing marketing copy, and auto-generating code.
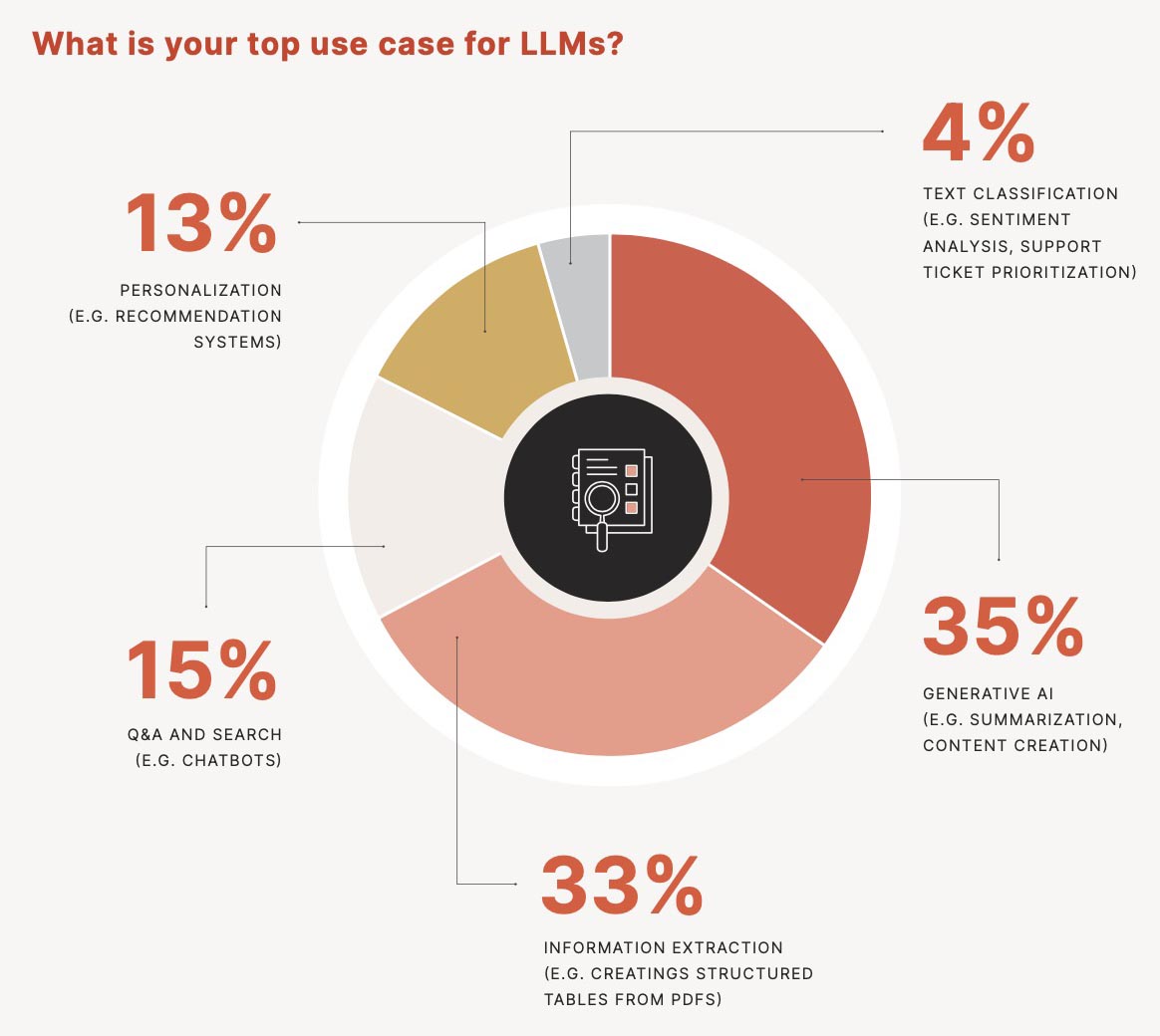
However, LLMs enable much more than generative tasks. The next most common use case, cited by 33%, is information extraction — using LLMs to convert unstructured data like PDFs or emails into structured tables for analysis. LLMs also have great potential for:
- Text classification: Analyzing sentiment, categorizing support tickets, moderating content
- Q&A and semantic search: Powering chatbots and internal knowledge bases
- Personalization: Generating tailored product and content recommendations
LLMs can be transformative across business functions. The key is customizing them with your own data to provide relevant, contextual insights.
But Significant Challenges Remain
While LLMs have incredible potential, significant obstacles are preventing many organizations from deploying them in production. When asked about their top challenge, 33% of survey respondents cited data privacy concerns with commercial LLM providers. Another 30% pointed to the high cost of training LLMs from scratch.
The complexity of grounding and fine-tuning LLMs with domain-specific data is another stumbling block. 46% of respondents said fine-tuning is too complex or they don’t know how to do it. Only 11% reported successfully fine-tuning an LLM with optimal results.
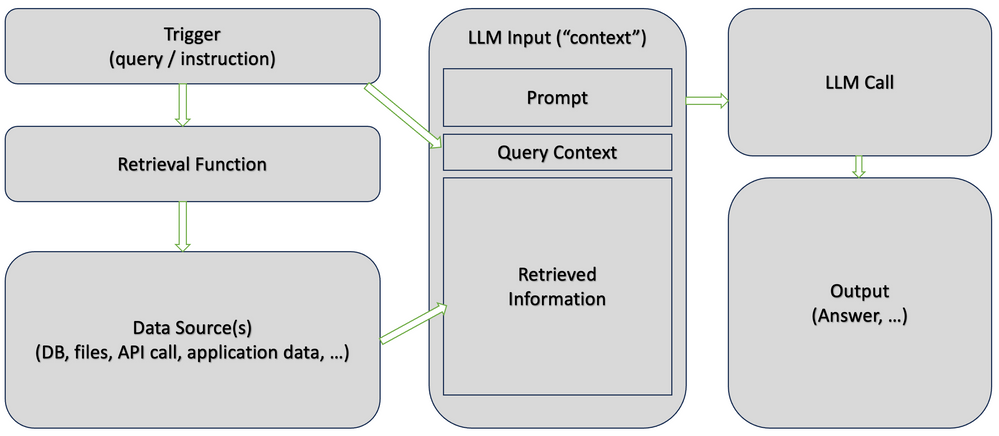
Other challenges include latency and throughput issues for real-time applications like chatbots, and LLMs’ tendency to “hallucinate” inaccurate or inconsistent outputs. “Today’s LLMs do a great job answering generic questions based on public data,” notes Predibase Chief Product Officer Devvret Rishi. “That’s 90% of the journey, but that last mile is where they’re struggling to provide contextual insightsthat are relevant to a business.”
The Rise of Open-Source LLMs
Strikingly, less than 25% of surveyed companies are comfortable deploying commercial LLMs in production, largely due to the data privacy and cost issues mentioned above. In response, many are turning to the booming open-source LLM ecosystem.
A leaked Google memo from May 2023 highlighted how open-source LLMs are rapidly closing the gap with commercial offerings. Even commercial players are jumping on the open-source bandwagon, like Meta‘s recent shift to open-sourcing their LLaMA models. Powerful open-source LLMs are now being released almost weekly from the research community.
Open-source LLMs allow organizations to retain control over their data by deploying the models in their own cloud environment. They also enable more customization to an organization’s specific use case and domain. “We see a massive opportunity for customized open-source LLMs to generate real-time insights across our large corpus of conservation project reports,” shares Dave Thau, Global Data and Technology Lead Scientist at WWF.
The Path Forward for Enterprises
So how should organizations prepare for the rise of LLMs? We have a few key recommendations:
- Establish acceptable use standards and a central “LLM office.” Ground rules should be put in place for what data can be used with LLMs and how outputs can be leveraged. A central team, including IT, legal, and other stakeholders, can help manage LLM use, establish best practices, and ensure standards are followed–especially in the early stages.
- Train employees on LLM quirks and prompt design. Everyone interacting with LLMs should understand their limitations, like hallucinations, and how to effectively query them. More advanced training on prompt engineering can further boost results.
- Break down data silos. Many LLM applications, like information extraction and retrieval, require access to data that may be scattered across organizations today. Identify high-value data sources and invest in making them accessible.
- Don’t rush to replace humans–focus on augmentation. While particular tasks may be automated with LLMs, most jobs are unlikely to be fully replaced anytime soon. Focus on using LLMs to augment employee capabilities and productivity vs. cutting headcount.
- Consider leveraging an LLM platform. If you have a strong development team, there could be an opportunity to build your own corporate LLM using open-source systems. Commercial options from Meta, OpenAI, or Google is another path. And independent offerings like Predibase (BTW they did not sponsor this article, we just used their survey results as a source) aim to simplify the complexity of deploying and customizing open-source LLMs for SMB companies.
The Future of Work
While the survey results surfaced both significant excitement and concerns around LLMs, most experts believe widespread job loss fears are overblown, at least in the near-term. The history of technology has shown that in the long run, new technologies create more jobs than they eliminate. For example, the invention of the tractor in 1892 made farming more efficent and allowed farmers to expand their work into new areas.
“LLMs are much more complicated to use effectively in an organizational context than is typically acknowledged,” notes Wharton School Professors Peter Cappelli, Prasanna Tambe, and Valery Yakubovich in MIT Sloan Management Review. “They have yet to demonstrate that they can satisfactorily perform all of the tasks that knowledge workers execute in any given job.” Rearranging work among existing employees to find excess positions that can be cut is unlikely to be easy or cost-effective.
Instead of wholesale replacement, LLMs seem much more likely to unbundle knowledge work into component tasks, some of which may be fully automatable, but many of which will simply be augmented by LLM-powered tools. Employees will increasingly collaborate with LLMs to enhance their productivity and capabilities.
The organizations that will gain the most from this shift are those that thoughtfully embrace this human-machine collaboration. By establishing responsible usage practices, enabling employee skill development, making information accessible, and leveraging platforms to efficiently operationalize the technology, enterprises can harness the full potential of LLMs while navigating the obstacles. Those that do will have a strong competitive advantage in the years ahead.
How Should Companies Get Ready for Large Language Models? Survey reveals how enterprises are adopting large language models, the top opportunities and challenges, and what it means for the future of work. #LLMs #FutureOfWork #LLMSurvey… Share on X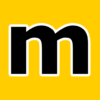
Leave a Reply